

Knowledge Base
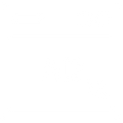
Central Hub for Ad Placement Experiment Insights
Project Type
End-to-end product design for a Rokt internal tool
My Role
Product Designer
Target Users
600 Rokt Employees
Duration
3 Months (Dec 2022 - Fec 2023)
Contribution
-
User research
-
Design
-
Prototypes
-
Product roadmap
-
User testing
Impact
50% of the Customer Success and Solutions team visits the Page Experiments Knowledge Base monthly
20% of new page experiments replicated/inspired by knowledge base
50% of these experiments see 5+% improvement in primary success metric
100% backfill of all analysis in the Vetted Experiments Register

Creative Experiments Table

Experiment Details Page
Overview
Background
At Rokt, our commitment to refining the user experience and maximizing the impact of ad placements led to the creation of an internal experimentation platform. This platform serves as a dynamic space for gradually implementing design and copy changes in our ad placements. Historically, decisions regarding these changes were made by customer success, account managers, and product teams based on assumptions, often without a thorough examination of past experiment data across various verticals and similar tests.

One Platform Experiment Reporting Tool
The Problem
There is no singular source of truth for insights derived from the outcome of Experiments.

Despite the evolution and expansion of our Experiments platform, a significant challenge emerged – the lack of a centralized repository for insights derived from experiment outcomes. As the platform's usage continues to surge, the ability to share and apply learnings becomes paramount to enhancing the value of our experimentation endeavors for clients.
Customer success teams face a critical need to...
-
Identify successful experiments
-
Understand the factors contributing to their success
-
Leverage these insights to deliver value to clients.
To address this challenge effectively, we must focus on alleviating three key pain points:
1. Discoverability of Experiments:
Locating past experiments proves challenging for Customer Success users who may not have a predefined search criteria. Enabling the ability to browse by vertical, sort by percentage uplift, or filter by specific experiment types enhances the user experience, guiding users in generating new experiments and providing valuable insights to clients.
2. Understanding Experiment Setups:
Context is crucial. Users need to comprehend the reasoning behind hypotheses, differences in variants, and the exclusion of other variants. Unquantifiable factors, such as the history of client relationships and macroeconomic influences, further complicate matters. Unraveling the "why" is pivotal for replicating success.
3. Interpreting Experiment Results:
The complexity of statistical concepts creates confusion, especially when determining the optimal decision. Many users grapple with confidently answering the question, "Was the most optimal decision made?" To instill confidence in decision-making and foster best practices over time, explicit, expert-vetted verification is essential.expert-vetted verification is needed to instill confidence in decision making, and in time, help form best-practices.
Types of Experiments
At Rokt, our mission is to enhance the relevancy and centralization of ads presented during the checkout experience. Within our marketplace, we cater to two distinct sides, each requiring specific types of experiments.
Creative Experiments
The Supply Side - Our Commerce Partners
-
These types of experiments test what the advertisers control
-
Users encounter offers from diverse advertisers during checkout, driven by machine learning to maximize relevance
-
Advertisers span various verticals and sub-verticals: Hulu, Disney, Paypal
-
Preliminary Types of Test Defined
-
CTA copy
-
Landing Page Link
-
Copy
-
Image
-
Page Experiments
The Demand Side - Our Advertisers
-
These experiments test what our e-commerce partners can control
-
The e-commerce site is the initial destination for users purchasing items or services
-
Notable partners: Uber, Ticketmaster, AMC Theaters
-
Preliminary Types of Test Defined
-
Design
-
Placement location
-
Types of offers shown
-
Type of placement i.e. overlay vs embedded
-

Example of a Rokt Overlay Placement on the Confirmation Page
Creative Experiments Table
Preiminary Requirements
Table Columns
-
Experiment name
-
Account name
-
The client
-
-
Verification status
-
Manual review to ensure conclusive and impactful results
-
-
Vertical
-
I.e. Expedia under travel vertical
-
-
Elements
-
Specifies what is being tested
-
-
Primary success metric
-
Uplift
-
Indicates how much better or worse variants performed against to the control
-
-
Duration
-
Date ended
-
Status
-
Probability to beat
-
The likelihood of an variant outperforming the control
-
Additional Requirements
-
The table should be filterable for the mentioned columns
-
Admin role responsible for tagging of experiment types, verification status, and adding comments
Card Sorting
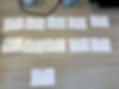
A card sorting exercise was conducted with 5 users to determine the best order of the columns.
V1 Low-Fi Designs

Option 1: Full Width Table With Elastic Search Filtering

Option 2: Dual Filter Panel & Table
Low-Fi A/B User Testing Round 1
I created a prototype and craft a usability testing study to gather feedback and iterate the creative experiments table and determine design & UX changes for the next iteration.
Participants
7 participants, mix of teams & vertical owners
Method
30 min moderated interviews / concept validation
Results
-
Users preferred the Option 2: filter sidebar better, as the full width table was overwhelming
-
Change elements & verticals to be clickable filters
-
Add sub verticals & pods
-
Uplift
-
Slider hard to use
-
Surface which variant won
-
-
Probability to beat hard to understand
-
Should be labelled i.e. "Very Good”
-
-
Add apply button before executing on filters
-
Experiments could be testing more than one thing - add multiple elements tags
-
Conversion rate per impression over referral rate is the most important metric and should be shown by default
-
"Element" for types of test ambiguous… change to test type
-
Add date range filter
-
Date range should be be relative
-
i.e. past 6 months vs manually entering a date
-
-
New test type sub categories (see below) i.e. if image is brand or logo
Disagree, Then Commit
A division arose within the team regarding the presentation and sorting of uplift in the Knowledge Base.
Initially, we presented uplift relative to the baseline and sorted it by the highest uplift. However, user feedback and a key issue I identified prompted a reconsideration of this approach.
Firstly, as an experiment can have one or more variants, the initial presentation did not distinctly convey which variant performed better or worse. Furthermore, the ambiguity in the range of the primary success metric, specifically in relation to a single variant, further complicated the understanding of experiment outcomes.
Secondly, default sorting by the highest uplift meant that only variants outperforming the control were surfaced at the top. Yet, valuable information about variants that performed worse than the control was not emphasized. Controls and variants represent different placements, so negative uplift in a control is still valuable information for Rokt employees.
In response to these challenges, I proposed four alternative options during the subsequent round of low-fi user testing to have data to define the best direction to move forward with and have team alignment.

The four uplift column options presented to users
During the next round of user testing, we aligned on the best option to move forward...
Low-FI Designs V2 & User Testing

Some Key Takeaways & Changes
1. Having uplift and prob to beat baseline next to each other added layer of confusion
Change: Move Prob to Beat to far right of table
2. Users thought blue link is experiment details, but was supposed to be OP link
Change: Remove blue link from table & add One Platform Link to Details Page
3. Users not sure what verified means
Change: Add banner at top explaining it
4. Users didn’t know what the prob to beat baseline category tags’ ranges were
Change: Add hover info icon tooltip in table
5. Filtering for Test Types
Users clicked the filter section opposed to the filter tokens at top. They aren’t in close proximity and caused confusion
Change: Make test types a dropdown filter & remove sub filter accordions
NOTE: For MVP, we weren’t able to implement all the test types
6. Users want percentage of traffic allocation to be filter
6. We added sub filters for test types i.e. if the CTA has the offer in it for not. But users preferred that these types of tests have their own tokens in the test type columns.
Change: Add Sub Test type
Not Shown in Above Mock
-
Users wanted experiment recommendations box to show when hovering over ended experiments
-
We added a number of variants in filter, but users were confused if the baseline is included in it or not
-
Change: Remove baseline from count and add info icon
-
-
Add created by filter, so employees can filter for their own experiments or other employees
Uplift
-
Most of users preferred option 1 sorted by absolute uplift
-
Users still wanted column to clearly represent negative or positive uplift
-
Users found the word absolute confusing though
-
Change: Just call it uplift
-
-
The options with percentages were confused because some thought it meant the percent a variant/basline is winning/loosing
New Creative Test Types
User sessions revealed that the initially identified creative test types were too narrow. After consolidating user feedback and conducting additional desk research, the following examples showcase users' preferences for more versatile filtering options.

MVP vs Post MVP Features
At this juncture, numerous feature requests had accumulated, but not all could be feasibly implemented by our OKR delivery deadline. Collaborating with my Product Manager, we distinguished features to be included in the MVP and those scheduled for later implementation. Subsequently, I conducted a workshop with a broader team to collectively assess and rank post-MVP features based on a scale of impact versus feasibility. These exercises allow me to aid in the roadmapping of Knowledge Base.

Final High-Fi MVP Designs

MVP Creatives Knowledge Base Table

Admin View
In Depth Discovery UX Research
At this stage, although we had received feedback on the Knowledge Base creatives table, numerous questions persisted.
Concrete requirements for page experiments within the Knowledge Base, especially in terms of defining experiment types, were lacking. Furthermore, we encountered a shortage of data supporting the specifications for the experiment details page, and the overall problem space for page experiments in the Knowledge Base remained unclear.
As highlighted earlier, our current users heavily rely on the legacy tool, One Platform, to manage experiments. Given this dependence, we aimed to uncover opportunities for improvements in that domain that could also be applied to the Knowledge Base.
In this UX research study, my role involved vigilant monitoring of user workflows, a deep dive into identified issues, clarification of problem areas, and the identification of opportunities and feature requests for both Knowledge Base and experimentation features within One Platform.
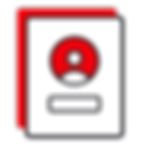
Participants
A diverse group of 14 individuals, encompassing roles in customer success, account management, and operations, providing insights across both creative and page experiments
Method
60 min 1-on-1 interviews
Research Questions
General
-
Who are the largest partners running experiments, and what are the reporting requirements for them?
-
How can we optimize the set up and tracking of experiments?
-
What pain points do users (CS, partners, and advertisers) currently encounter regarding experiments?
-
-
What information do users need from previous experiments to make better informed decisions about future ones?
-
Which network wide insights do we need to convey?
-
How can we prevent inconclusive experiments?
-
-
If an experiment is inconclusive, what information do users need to understand why it is?
-
What tools are employees using outside OP that can be integrated into OP or Knowledge Base
Creative Experiments Knowledge Base
-
Which reporting metrics and graphs are beneficial?
-
How do users want to micro categorize elements?
-
I.e. Creative text can be split up into body text and header
-
-
How can we efficiently display & compare variant results for each experiment?
-
How do users want to compare variant results across different experiments?
-
What additional features do users want?
-
How do users want to tag and filter experiments to easily find and compare them?
-
What additional information and metrics do we need to convey?
Page Experiments Knowledge Base
-
What information is critical when interpreting experiment results, and how is this difficult in OP?
-
From an overview and granular standpoint
-
-
How do users want to categorize page experiments, and how can we efficiently display it?
-
How do users want to compare different page experiments and variants?
-
How can we optimize the discovery of page experiments?
-
Which filters are valuable?
-
One Platform
-
What is the full end-to-end user journey?
-
What pain points do users encounter?
-
Which features do users want to add?
-
Which other services and tooling are people using?
-
-
How can we optimize, centralize, and streamline the creation and tracking of experiments?
-
How do account managers want to add items in a queue for ops to review in OP?
-
How does Ops want to review items efficiently?
-
-
How can we optimize the tracking and reporting of experiments?
-
For each experiment and holistically
-
-
How can we prevent inconclusive experiments and add preventative measures?
Medical Leave of Absence... Couldn't Finish Research :(
Unfortunately, as I launched this research project, I faced a severe medical condition, leading to a necessary short-term medical leave spanning a few months.
Before my departure, I conducted 11 interviews, synthesized insights from some of them, began categorizing page experiments, and initiated the end-to-end user journey for experimental work. Some of these insights laid the foundation for the subsequent design work on the Knowledge Base, specifically the creative experiments details page.
When I returned from my leave, I was switched projects.

User Journeys

Creative Experiments Details Page
While initiating the research study, I simultaneously commenced work on the Knowledge Base creative experiment details page. This page is designed to allow users to delve deeper into a creative experiment, enabling functionalities such as result analysis filtering, segmentation (by age, gender, etc.), access to experiment recommendations, placement previews, and more.
Design Critique & User Feedback of One Platform Experiment Details Page

1. The Progress Bar
The progress bar used to convey the probability to beat is highly misleading, particularly for first-time users. Its prominent appearance may quickly lead them to associate it with the variant that performed the best for the selected metric, and the progress bar is not always an accurate representation of the probability to beat categories.
Example: A variant might show the highest probability to beat in the progress bar, creating an impression of significant success. However, in reality, it may still fall within the "Even with Baseline" category, causing a misalignment between user expectations and the actual outcome.
2. Relative Metrics
Experiment data is limited to only relation to baseline. Since users like absolute uplift, are also interested in seeing the variants compared against the best or worst creatives in total- including baseline and variants.
3. Success Metrics Tabs not as Important as Segmentation
The quick change mode view is for the success metrics, when usually there is a most important one and users use the segmentation more frequently. To access segmentation, it is 2 clicks instead of one.
4. Winning Indicators & Sort Order
The experiment results table lacks clear indicators of the order in which the variants won- the variants are always below the baseline. Additionally, by default it's always baseline and then variants below in numerical order.

5. Segmentation
Iit is always ordered in chronological order with baseline at top. Users need to manually skim the page to quickly see which segment won in total between all variants & baseline. Additionally, it’s hard to know which variant or baseline one per segment. There also isn’t a clear standing out winner.
Additional Notes
-
Users don't have a easy way to preview the baseline creative in this view, it's in an other page
-
Additionally, it's a lot of clicks because they need to be opened up individually
-
- There are no previews of the variants possible anywhere in One Platform
- Lacking start date, which is more important than end date
V1 Low-Fi Mocks

Default Landing

Segmentation

Preview Creatives Modal
User Testing Feedback
-
Liked
-
Ranked from best performing to worst
-
Having the progress bar for success metric performance than probability to beat
-
Relativity to best & worst and keeping this default
-
Ability to easily preview creatives
-
Having group by all segments default & the no grouping option
-
Tabs for segments vs success metrics
-
-
Didn't like
-
Having the variant name to the right of success metric results
-
Because of the variants naming conventions, it's a quick identifier of what it is without seeing the preview
-
-
Having impressions on far right to the table
-
-
Pain Points
-
User found it hard to find the ability to change relativity to baseline since the selectors don't have labels and it was hidden over a brand new concept
-
The colored tokens for baseline & variants don't accurately represent what is changed between them
-
Forward and backwards arrow keys to navigate creative previews are not prominent enough
-
Some users found it difficult to find the group segments by selector
-
Test type not that prominent
-
-
Wants
-
Clicking the recommendations box on different segments etc. to manipulate the view/data
-
Surface the sub test categories and their values i.e. Offer in header vs not
-
During segmentation no groupings and group by segment mode, to view which segment has the biggest uplift as a percentage of the overall experiment impressions
-
V2 Hi-Fi Mocks
.png)
Default Landing

Segmentation: Group By Segment

Segmentation: Combine All

Preview Creatives Modal